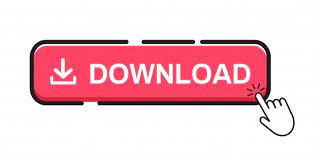
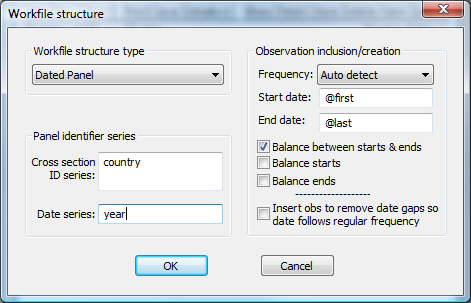
- HOW TO MOVE DATA IN EVIEWS 10 HOW TO
- HOW TO MOVE DATA IN EVIEWS 10 INSTALL
- HOW TO MOVE DATA IN EVIEWS 10 MANUAL
- HOW TO MOVE DATA IN EVIEWS 10 CODE
- HOW TO MOVE DATA IN EVIEWS 10 SERIES
'restructure the page to be based on this day count rather than actual dates 'contract the page so that days before the second recorded death in each country are removed
HOW TO MOVE DATA IN EVIEWS 10 SERIES
This series is equal to 0 if the number of deaths on a date is equal to the minimum number of deaths for that country (nearly always 0, but for China, the data starts after the first recorded death), and then counts up by one for dates after the minimum. Pagecontract if a series containing the number of days since the first death was recorded in each country. 'contract the page to only include countries with greater than 160 deaths 'copy the deaths series to the panel pageĬopy(c=sum) stack\deaths * country country
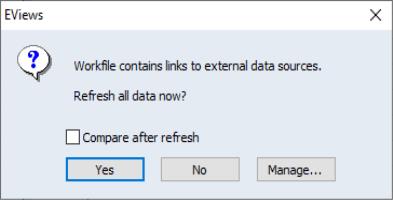
Pagestruct province country the original page 'do some renaming and make the date series 'import the death data from Johns Hopkins
HOW TO MOVE DATA IN EVIEWS 10 CODE
The code to produce this graph, including importing the death data from Johns Hopkins is: The graph shows that for most countries the growth rate of deaths (approximated by using log-scaling) is increasing, but at a slower rate. The first is a graph showing the 3 day moving average of the number of deaths per day since the first death was recorded in a country, for countries with a current number of deaths greater than 160:
HOW TO MOVE DATA IN EVIEWS 10 HOW TO
IMF.As a follow up to our previous blog entry describing how to import Covid-19 data into EViews and produce some maps/graphs of the data, this post will produce a couple more graphs similar to ones we've seen become popular across social media in recent days.
HOW TO MOVE DATA IN EVIEWS 10 MANUAL
2001 Quarterly National Accounts Manual - Concepts, Data Sources, and Compilation. title( "Chow-Lin interpolation: \nannual sum of benchmarked = benchmark", fontsize = 14)įor more information on the pyeviews package, including a list of functions, please take a look at our whitepaper on the subject. plot( benchmark, 'ro', label = 'benchmark')Īx2.
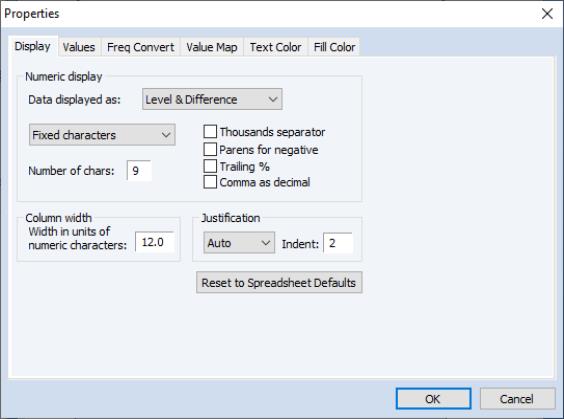
set_ylabel( 'indicator & interpolated values', color = 'b')Īx2. # multiply the indicator series by 10 to put it on the same axis as the benchmarked series ax1. plot( benchmarked_reindexed, 'b-', label = 'benchmarked') pyplot as plt > # reindex the benchmarked series to the end of the quarter so the dates match those of the indicator series benchmarked_reindexed = pa. > # load the matplotlib package to plot import matplotlib. We'll call the annual series "benchmark" and the quarterly series "indicator":
HOW TO MOVE DATA IN EVIEWS 10 INSTALL
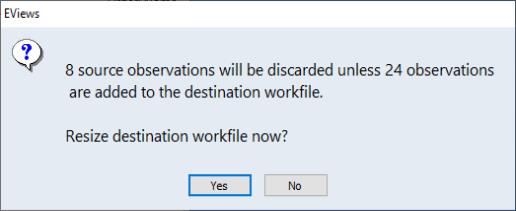
The quarterly interpolated series is chosen to match the annual benchmark series in one of four ways: first (the first quarter value of the interpolated series matches the annual series), last (same, but for the fourth quarter value), sum (the sum of the first through fourth quarters matches the annual series), and average (the average of the first through fourth quarters matches the annual series). It has the ability to use a higher-frequency series as a pattern for the interpolated series to follow. Chow-Lin interpolation is a regression-based technique to transform low-frequency data (in our example, annual) into higher-frequency data (in our example, quarterly). We're going to use the popular Chow-Lin interpolation routine in EViews using data created in Python. Here's a simple example going from Python to EViews. (For more information on COM and EViews, take a look at our whitepaper on the subject.) This package uses COM to transfer data between Python and EViews. The purpose of the pyeviews package is to make it easier for EViews and Python to talk to each other, so Python programmers can use the econometric engine of EViews directly from Python.
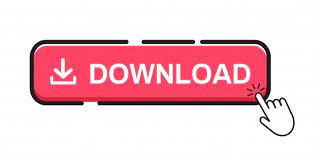